Abstract
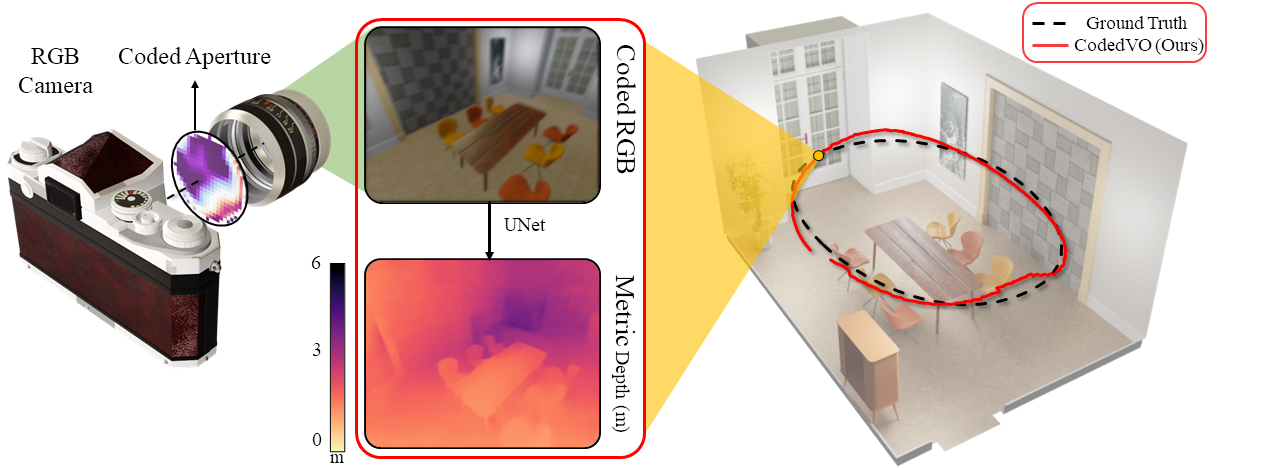
Figure: Our proposed approach leverages coded aperture to predict metric dense depth maps using only an RGB sensor tailored for monocular odometry estimation.
Autonomous robots often rely on monocular cameras for odometry estimation and navigation. However, the inherent challenge of scale ambiguity in monocular visual odometry remains a critical bottleneck. In this paper, we present CodedVO, a novel monocular visual odometry method that leverages optical constraints from coded apertures to resolve scale ambiguity. By integrating RGB and predicted metric depth using optical constraints, we achieve state-of-the-art performance in monocular visual odometry with a known scale. We evaluate our method in diverse indoor environments and demonstrate its robustness and adaptability. We achieve 0.08m average trajectory error in odometry evaluation in standard indoor odometry datasets.
Drawing inspiration from the evolution of eyes and pupils, researchers have developed coded apertures tailored for monocular camera systems. These aperture masks enable metric dense depth estimation from a single view by utilizing depth cues from defocus. However, these computational imaging methods are underutilized and not well-explored in the field of robot autonomy. This paper introduces \textbf{CodedVO}, a novel visual odometry method that leverages the metric depth maps from the geometrical constraints of a coded camera system and achieves state-of-the-art monocular odometry performance.
Paper
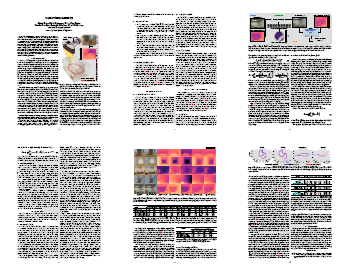 |
Sachin Shah*, Naitri Rajyaguru*, Chahat Deep Singh, Cornelia Fermüller, Christopher Metzler, Yiannis Aloimonos.
* Equal Contribution
|
|