An overview of our approach: The proposed geometric model is used to generate synthetic images which are further fed into a VSAIT to enable image-to-image translation. We then combine the synthetic data with real data to train an Unet for olive detection.
Modern robotics has enabled the advancement in yield estimation for precision agriculture. However, when applied to the olive industry, the high variation of olive colors and their similarity to the background leaf canopy presents a challenge. Labeling several thousands of very dense olive grove images for segmentation is a labor-intensive task. This paper presents a novel approach to detecting olives without the need to manually label data. In this work, we present the world's first olive detection dataset comprised of synthetic and real olive tree images. This is accomplished by generating an auto-labeled photorealistic 3D model of an olive tree. Its geometry is then simplified for lightweight rendering purposes. In addition, experiments are conducted with a mix of synthetically generated and real images, yielding an improvement of up to 66% compared to when only using a small sample of real data. When access to real, human-labeled data is limited, a combination of mostly synthetic data and a small amount of real data can enhance olive detection.
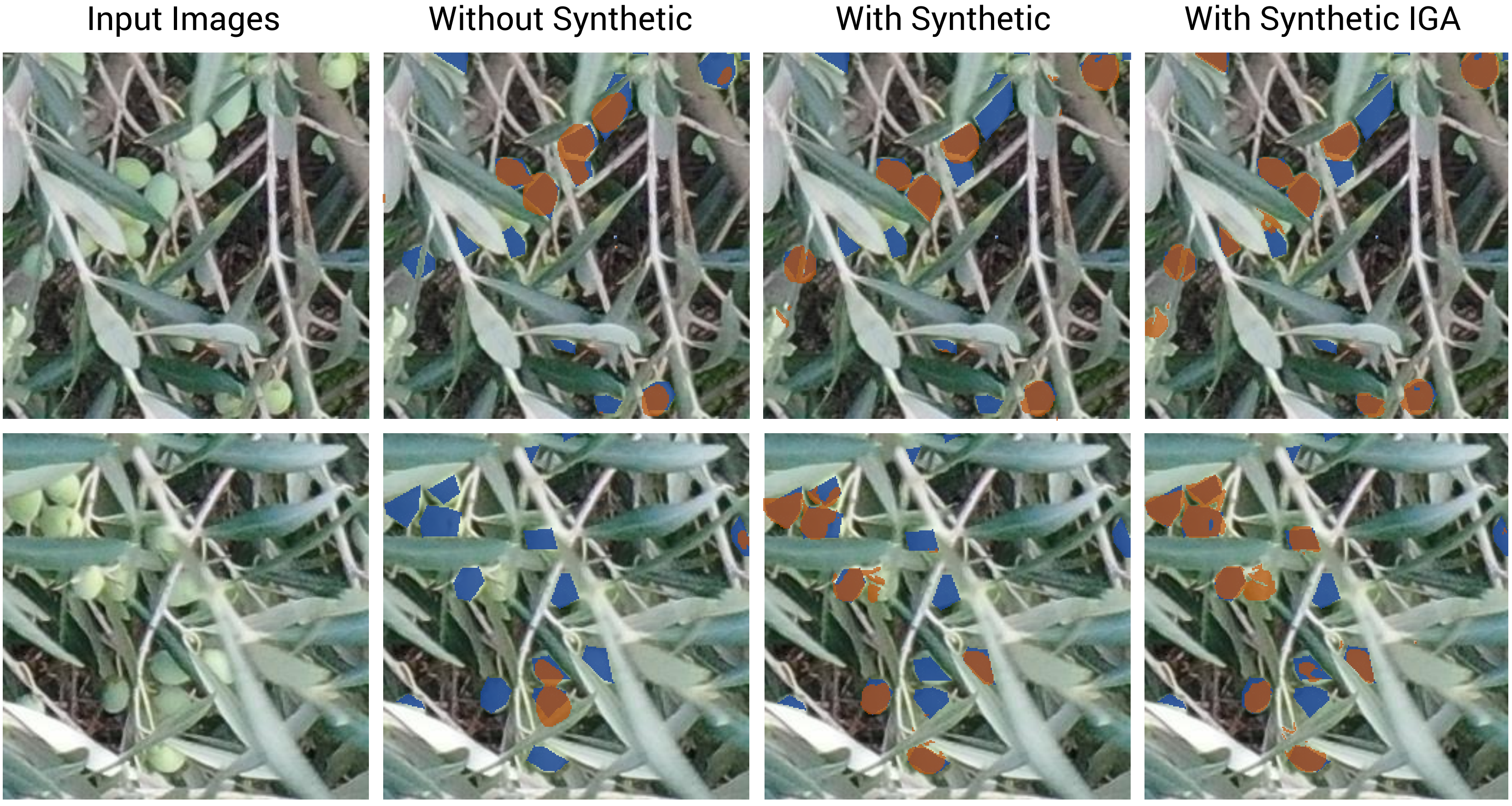
Each column left to right: Input image, prediction using only
real data, prediction using real and synthetic data, prediction using real and
synthetic data in IGA. Predictions are shown in orange and ground truths
are shown in blue. Adding synthetic data to the training set increases the
number of correct predictions.