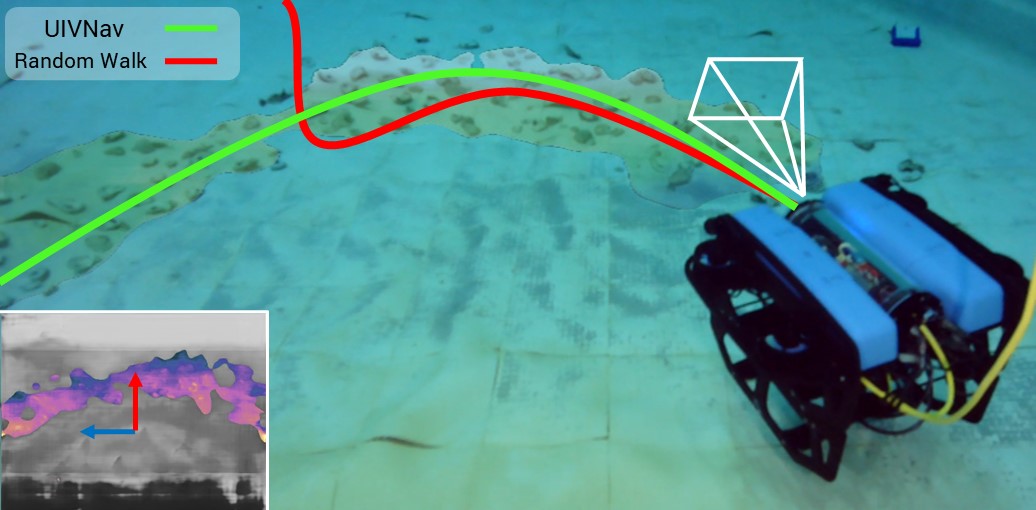
Figure 1: Example of two trajectories of BlueROV surveying oyster-reef
in a pool. On the bottom left is the current frame in the intermediate
representation that the robot observes.
Abstract
Autonomous navigation in the underwater environment is challenging due to limited visibility, dynamic
changes, and the lack of a cost-efficient accurate localization
system. We introduce UIVNAV, a novel end-to-end underwater
navigation solution designed to drive robots over Objects of
Interest (OOI) while avoiding obstacles, without relying on
localization. UIVNAV uses imitation learning and is inspired
by the navigation strategies used by human divers who do
not rely on localization. UIVNAV consists of the following
phases: (1) generating an intermediate representation (IR),
and (2) training the navigation policy based on human-labeled
IR.
By training the navigation policy on IR instead of raw
data, the second phase is domain-invariant, the navigation
policy does not need to be retrained if the domain or the
OOI changes. We show this by deploying the same navigation
policy for surveying two different OOIs, oyster and rock reefs,
in two different domains, simulation, and a real pool. We
compared our method with complete coverage and random
walk methods which showed that our method is more efficient
in gathering information for OOIs while also avoiding obstacles.
The results show that UIVNAV chooses to visit the areas with
larger area size of oysters or rocks with no prior information
about the environment or localization. Moreover, a robot using
UIVNAV compared to complete coverage method surveys on
average 36% more oysters when traveling the same distances.
We also demonstrate the feasibility of real-time deployment of
UIVNAV in pool experiments with BlueROV underwater robot
for surveying a bed of oyster shells
Paper
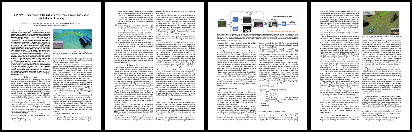 |
Xiaomin Lin, Nare Karapetyan, Kaustubh Joshi, Tianchen Liu, Nikhil Chopra, Miao Yu, Pratap Tokekar, Yiannis Aloimonos
|
|