In the era of deep learning, data is the
critical determining factor in the performance of neural
network models. Generating large datasets suffers from
various difficulties such as scalability, cost efficiency and
photorealism. To avoid expensive and strenuous dataset
collection and annotations, researchers have inclined towards
computer-generated datasets. Although, a lack of photorealism
and a limited amount of computer-aided data, has bounded the
accuracy of network predictions.
To this end, we present WorldGen - an open source
framework to autonomously generate countless structured and
unstructured 3D photorealistic scenes such as city view, object
collection, and object fragmentation along with its rich ground
truth annotation data. WorldGen being a generative model gives
the user full access and control to features such as texture,
object structure, motion, camera and lens properties for better
generalizability by diminishing the data bias in the network.
We demonstrate the effectiveness of WorldGen by presenting
an evaluation on deep optical flow. We hope such a tool can
open doors for future research in a myriad of domains related
to robotics and computer vision by reducing manual labor and
the cost of acquiring rich and high-quality data.
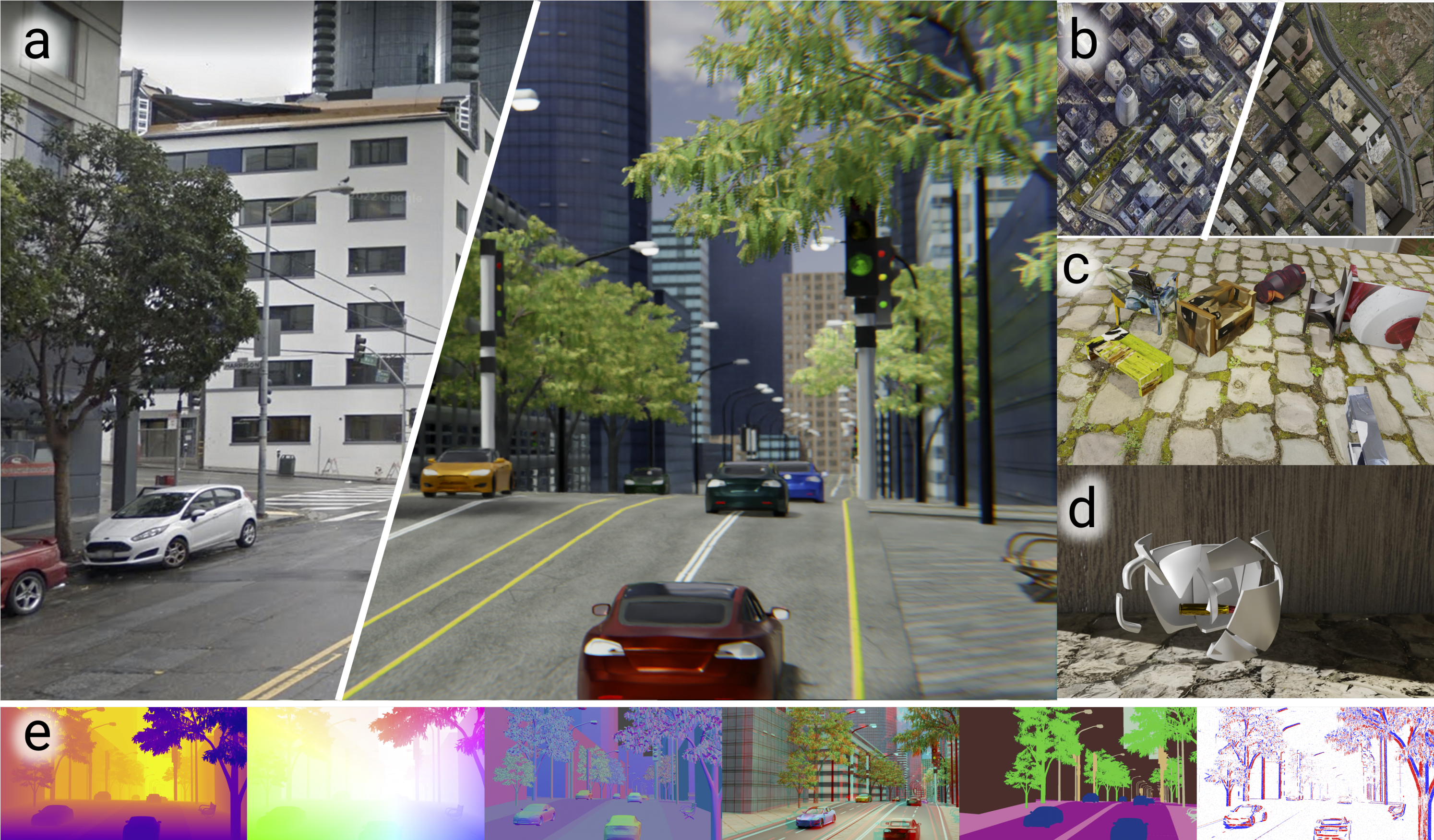
Fig. 1: Generative ability of WorldGen: (a) Comparison between Google
Street View (left) and the same street in WorldGen (right), (b) Comparison
of Google Maps satellite image vs. WorldGen top view, (c) Collection of 3D
objects in motion, (d) Object fragmentation,(e) Annotation from left to right:
depth, optical flow, surface normals, stereo anaglyph, image segmentation,
event frame.