Recent advances in object segmentation have demonstrated that deep neural networks excel at object segmentation for specific classes in color and depth images. However, their performance is dictated by the number of classes and objects used for training, thereby hindering generalization to never seen objects or zero-shot samples. To exacerbate the problem further, object segmentation using image frames rely on recognition and pattern matching cues. Instead, we utilize the 'active' nature of a robot and their ability to 'interact' with the environment to induce additional geometric constraints for segmenting zero-shot samples. In this paper, we present the first framework to segment unknown objects in a cluttered scene by repeatedly 'nudging' at the objects and moving them to obtain additional motion cues at every step using only a monochrome monocular camera.We call our framework NudgeSeg. These motion cues are used to refine the segmentation masks. We successfully test our approach to segment novel objects in various cluttered scenes and provide an extensive study with image and motion segmentation methods. We show an impressive average detection rate of over 86% on zero-shot objects.
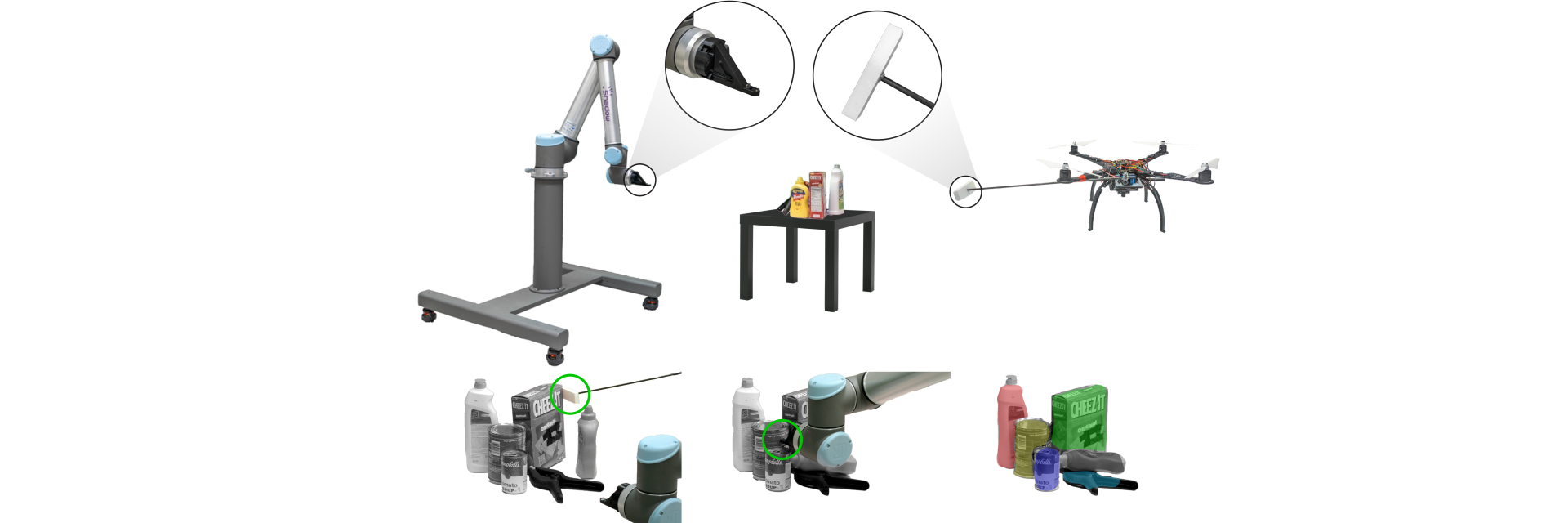
Figure: Top row: Robots (UR-10 and a quadrotor) used to physically interact (or nudge) with the objects to get motion cues for segmenting objects in a clutter. Bottom row (left to right): Initial Configuration of a cluttered scene and the first nudge being invoked, final nudge is invoked, final Segmentation of the cluttered scene. Green circles show the nudge operation.