Odometry on aerial robots has to be of low latency
and high robustness whilst also respecting the Size, Weight,
Area and Power (SWAP) constraints as demanded by the
size of the robot. A combination of visual sensors coupled
with Inertial Measurement Units (IMUs) has proven to be the
best combination to obtain robust and low latency odometry
on resource-constrained aerial robots. Recently, deep learning
approaches for Visual Inertial fusion have gained momentum due
to their high accuracy and robustness. However, the remarkable
advantages of these techniques are their inherent scalability
(adaptation to different sized aerial robots) and unification (same
method works on different sized aerial robots) by utilizing
compression methods and hardware acceleration, which have
been lacking from previous approaches.
To this end, we present a deep learning approach for visual
translation estimation and loosely fuse it with an Inertial sensor
for full 6 DoF odometry estimation. We also present a detailed
benchmark comparing different architectures, loss functions and
compression methods to enable scalability. We evaluate our
network on the MSCOCO dataset and evaluate the VI fusion
on multiple real-flight trajectories
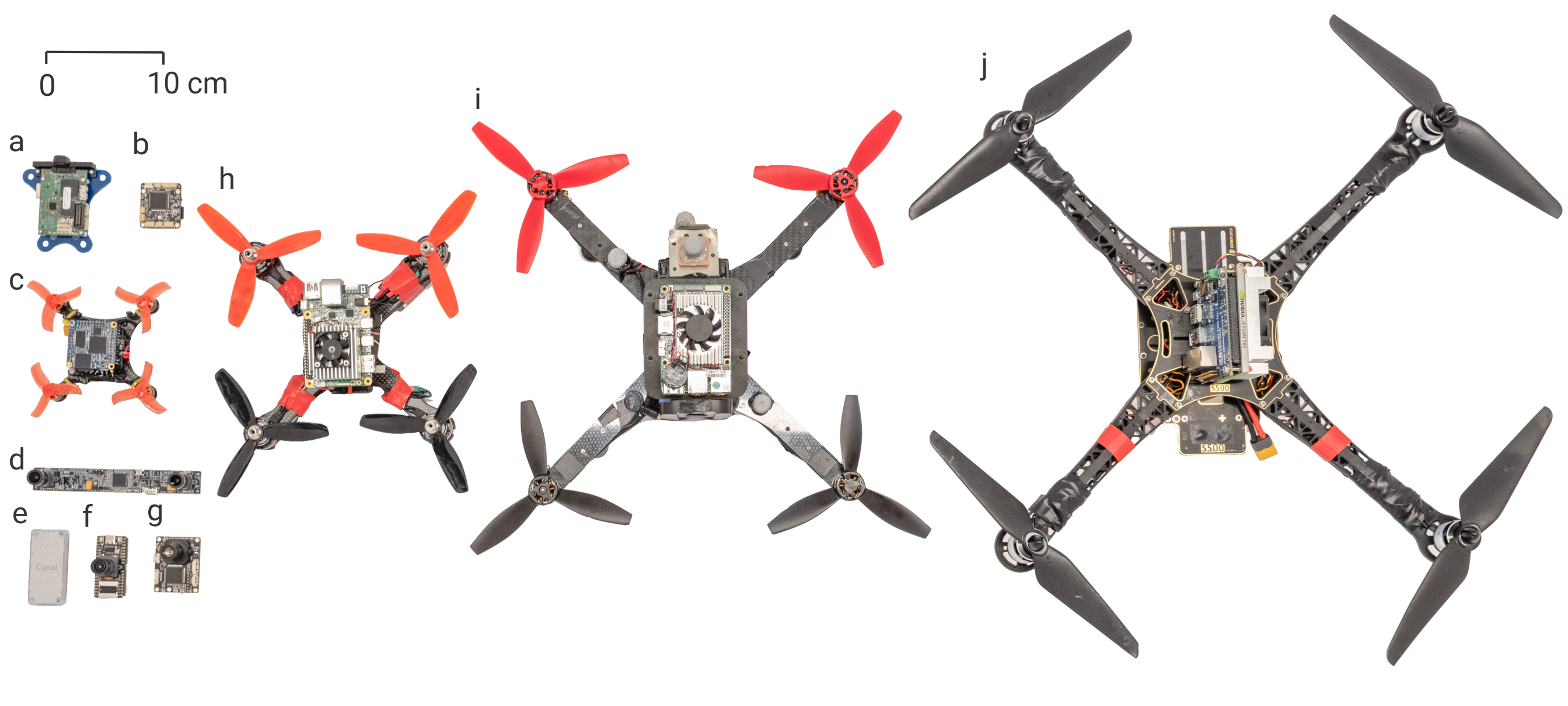
Figure: Size comparison of various components used on quadrotors. (a) Snapdragon Flight, (b) PixFalcon, (c) 120 mm quadrotor platform with NanoPi Neo Core 2, (d) MYNT EYE stereo camera, (e) Google Coral USB accelerator, (f) Sipeed Maix Bit, (g) PX4Flow, (h) 210 mm quadrotor platform with Coral Dev board, (i) 360 mm quadrotor platform with Intel Up board, (j) 500 mm quadrotor platform with NVIDIA Jetson TX2. Note that all components shown are to relative scale. All the images in this paper are best viewed in color.